
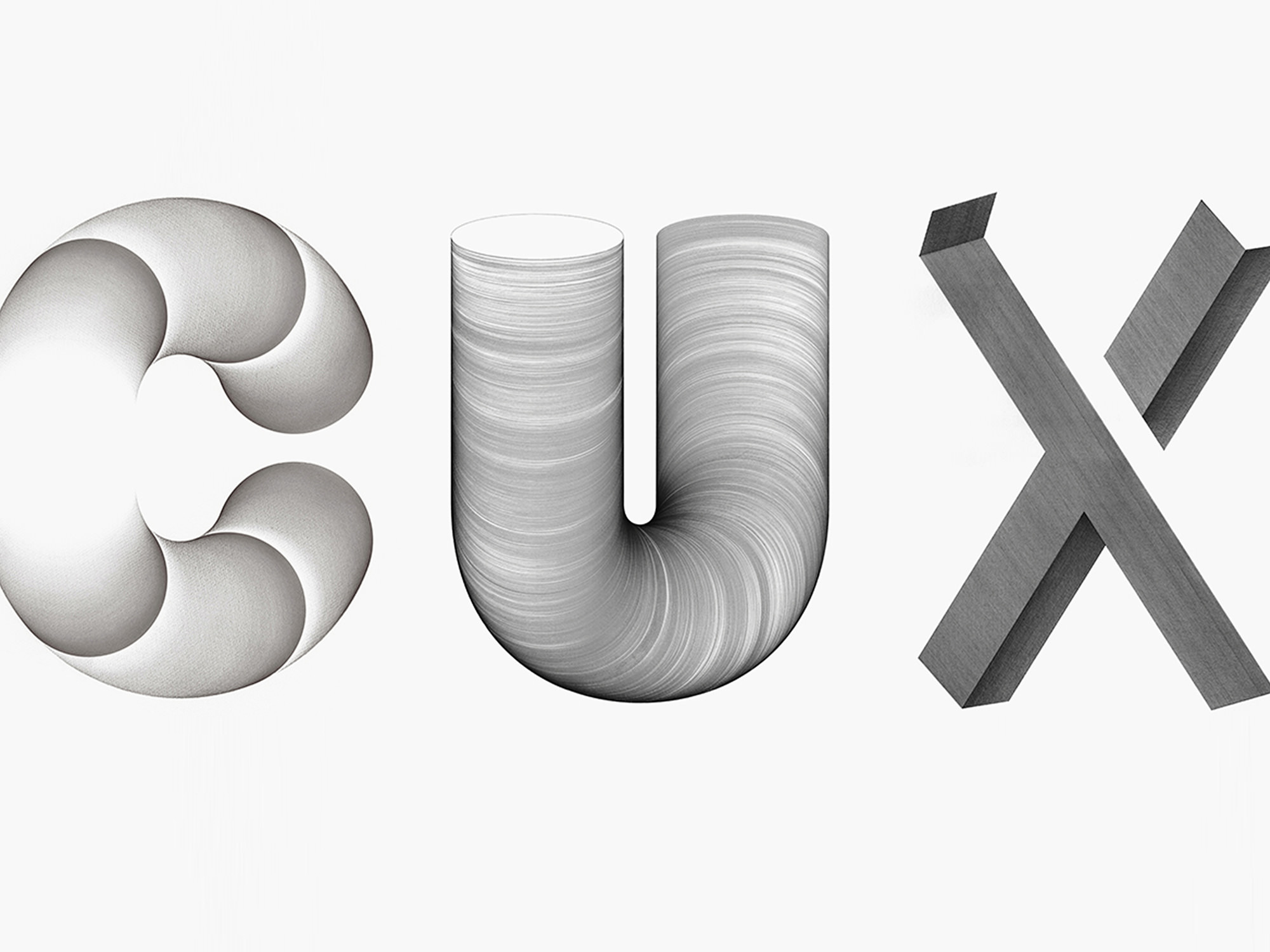
Although these machines can produce highly informative data from multiple samplings due to the non-invasive nature of hyperspectral imaging, there are significant drawbacks that have severely limited their accessibility to many plant researchers.
#Uncontrolled types by plotter drawing software#
The machine is controlled by software that accepts user input such as positional and instrument control commands. In these machines, the imaging instrument is typically mounted to an optimal point and a motor-driven axis moves the plants that need to be scanned, or vice versa. Recently, stationary machines have also been created to scan non-moving fields or targets. The widest use of hyperspectral imaging is from sensors mounted on drones or small aircraft. To alleviate these setbacks, researchers and private companies have developed machines to automate most if not all of the imaging process. These become important issues for the analysis of plant responses which tend to change rapidly in response to environmental or biotic stressors (requiring time-course data collection) and also to vary widely between different plant species or genotypes (requiring sampling from many individuals). For example, scheduling collections for multiple spectroscopic measurements across many samples and over many time points is often logistically difficult and even prohibitively time consuming, especially in the field where variable light conditions affect measurements. While such spectroscopic imaging techniques offer huge potential, they also raise many practical issues that currently limit applications. These spectra remain a rich resource information yet to be mined for plant studies and phenotyping, with the potential for many additional features of plant physiology and chemistry to be extracted. Some spectral features are known to be associated with specific chemical or stress responses, such as the detection of plant physiological stress using the photochemical reflectance index. For example, measurement of absorbance and reflectance features in the visible spectrum and out into the infrared (~ 400 to 2500 nm) have been used to directly estimate foliar structure, plant chemical composition, water content, and metabolic status. The technique uses variations in leaf optical properties that arise from the interaction of light and chemical bonds.
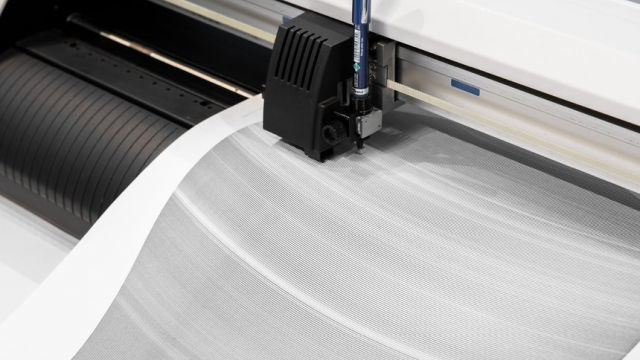

Reflectance spectroscopy is rapidly emerging as a highly effective and practical approach for the rapid, non-destructive estimation of a wide variety of chemical, biophysical, and metabolic plant traits in living tissue. Remote monitoring of plant physiology and biochemistry holds enormous potential for understanding plant growth and development in settings ranging from the laboratory to the field.
